Project Description
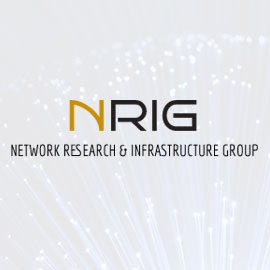
Scientific workflows are now being used in a number of scientific domains, including astronomy, bioinformatics, climate modeling, earth science, civil engineering, physics, and many others. Unlike monolithic applications, workflows often run across heterogeneous resources distributed across wide area networks. Some workflow tasks may require high performance computing resources, while others can run efficiently on high throughput computing systems. Workflows also access data from potentially different data repositories and use data, often represented as files, to communicate between the workflow components. Because of these data access patterns, the performance of networks and storage devices greatly influence how smoothly and quickly a workflow runs.
The Panorama project aims to address workflow performance through a three-pronged approach that involves: 1) developing analytical models that can predict the behavior of complex, data-aware scientific workflows executing in extreme-scale infrastructures; 2) determining what monitoring information and information analysis is needed to predict performance and detect anomalies in scientific workflow execution; and 3) discovering how to adapt the workflow execution and the infrastructure to achieve the potential performance predicted by the models.
Workflow performance will be studied using two Department of Energy applications that depend on workflows: Climate and Earth System Modeling (CESM), which processes large amounts of community data; and Spallation Neutron Source (SNS), which produces rich experimental data used in a variety of complex analyses.
RENCI employs analytical performance models and monitoring information to facilitate detection and diagnosis of performance anomalies, to manage resources, and to adapt workflows as needed. The RENCI team will use models to predict expected application behavior, and combined with correlated monitoring information, will develop algorithms to automatically detect anomalies in system behavior and to automatically diagnose the most likely cause(s) of any found anomalies. An analysis capability will correlate workflow monitoring information with resource performance measurements to provide a better understanding of which resources contributed to an observed behavior.
Partners:
- University of Southern California
- Lawrence Berkeley Laboratory
- Oak Ridge National Laboratory
- RENCI
- Rensselaer Polytechnic Institute
Funding:
Project Publications
- Anomaly Detection for Scientific Workflow Applications on Networked Clouds
- Enabling Persistent Queries for Cross-aggregate Performance Monitoring
- PANORAMA: An Approach to Performance Modeling and Diagnosis of Extreme Scale Workflows
- Toward an End-to-end Framework for Modeling, Monitoring and Anomaly Detection for Scientific Workflows
- Toward Prioritization of Data Flows for Scientific Workflows Using Virtual Software Defined Exchanges