Project Description
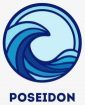
PosEiDon aims to advance the knowledge of how simulation and machine learning (ML) methodologies can be harnessed and amplified to improve DOE’s computational and data science. PosEiDon will provide an integrated platform that helps facility operators and scientists improve the overall end-to-end science workflow by (1) predicting the performance of complex workflows; (2) detecting and classifying infrastructure and workflow anomalies and “explaining” the sources of these anomalies; and (3) suggesting performance optimizations.
Partners: University of Southern California (Lead), Lawrence Berkeley Laboratory, Argonne National Laboratory, RENCI
Funding: US Department of Energy
Project Publications
- Anomaly Detection in Scientific Workflows using End-to-End Execution Gantt Charts and Convolutional Neural Networks
- End-to-end online performance data capture and analysis for scientific workflows
- Graph Neural Network for Anomalies Detection in Scientific Workflows
- Workflow Anomaly Detection with Graph Neural Networks
- Graph Neural Networks for Detecting Anomalies in Scientific Workflows
- Flow-Bench: A Dataset for Computational Workflow Anomaly Detection
- Experimenting TCP Performance with Fabric
- Elephants Sharing the Highway: Studying TCP Fairness in Large Transfers over High Throughput Links
- (POSTER) Network Testbed for Experimenting With Decentralized Federated Learning
- Advancing Anomaly Detection in Computational Workflows with Active Learning
- Large Language Models for Anomaly Detection in Computational Workflows: from Supervised Fine-Tuning to In-Context Learning