Project Description
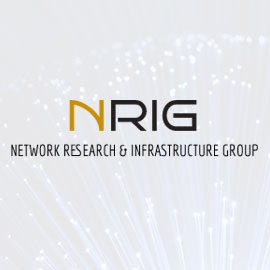
Data-driven science applications often depend on the integrity of the underlying scientific computational workflow and on the integrity of the associated data products. However, experience with executing numerous scientific workflows in a variety of environments has shown that workflow processing suffers from data integrity errors when workflows are executing on national cyberinfrastructure (CI). These errors can stem from failures and unintentional corruption at various layers of the system software and hardware. However, today, there is a lack of tools that can collect and analyze integrity-relevant data while workflows are executing and thus, many of these errors go undetected and corrupt data becomes part of the scientific record. The goal of this proposed work is to automatically detect, diagnose, and pinpoint the source of unintentional integrity anomalies in scientific workflows executing on distributed CI. The approach is to develop an appropriate threat model and incorporate it in an integrity introspection, correlation and analysis framework that collects application and infrastructure data and uses statistical and machine learning (ML) algorithms to perform the needed analysis. The framework will be powered by novel ML-based methods developed through experimentation in a controlled testbed and validated in and made broadly available on NSF production CI. The solutions will leverage and be integrated into the Pegasus workflow management system, which is already used by a wide variety of scientific domains. An important part of the project will be the engagement with selected science application partners in gravitational-wave physics, earthquake science, and bioinformatics to deploy the analysis framework for their workflows, and iteratively fine tune the threat models, the testbed, ML model training, and ML model validation in a feedback loop.
The proposed technologies will result in increased confidence in the veracity of workflow-based scientific results. The novel ML-based techniques for offline and online analysis of integrity data from various sources will contribute to the knowledge of what types of ML techniques work well for anomaly detection and fault attribution in distributed systems. The proposed anomaly injection framework, the Chaos Jungle, will enable other computer scientists to test their anomaly detection algorithms. CI designers will also be able to use the Chaos Jungle to test the robustness of their infrastructure. Although Pegasus is the target workflow management system for delivering the proposed integrity introspection capabilities, the methods will be reusable by other workflow and data management systems and will be available to scientists to run workflows on other CI such as campus clusters, clouds or leadership class facilities. Through close interactions with application partners, and evaluation of proposed analysis methodologies and ML models on NSF production CI, this work will increase assurances of the integrity of scientific workflows while maintaining ease of use and flexibility to allow for use across a variety of science domains and cyberinfrastructures.
Project partners:
- Renaissance Computing Institute (RENCI) (Lead)
- University of Southern California Information Sciences Institute (USC/ISI)
- Indiana University
Related links:
The IRIS Project is supported by the National Science Foundation under Grant 1839900. The views expressed do not necessarily reflect the views of the National Science Foundation or any other organization.